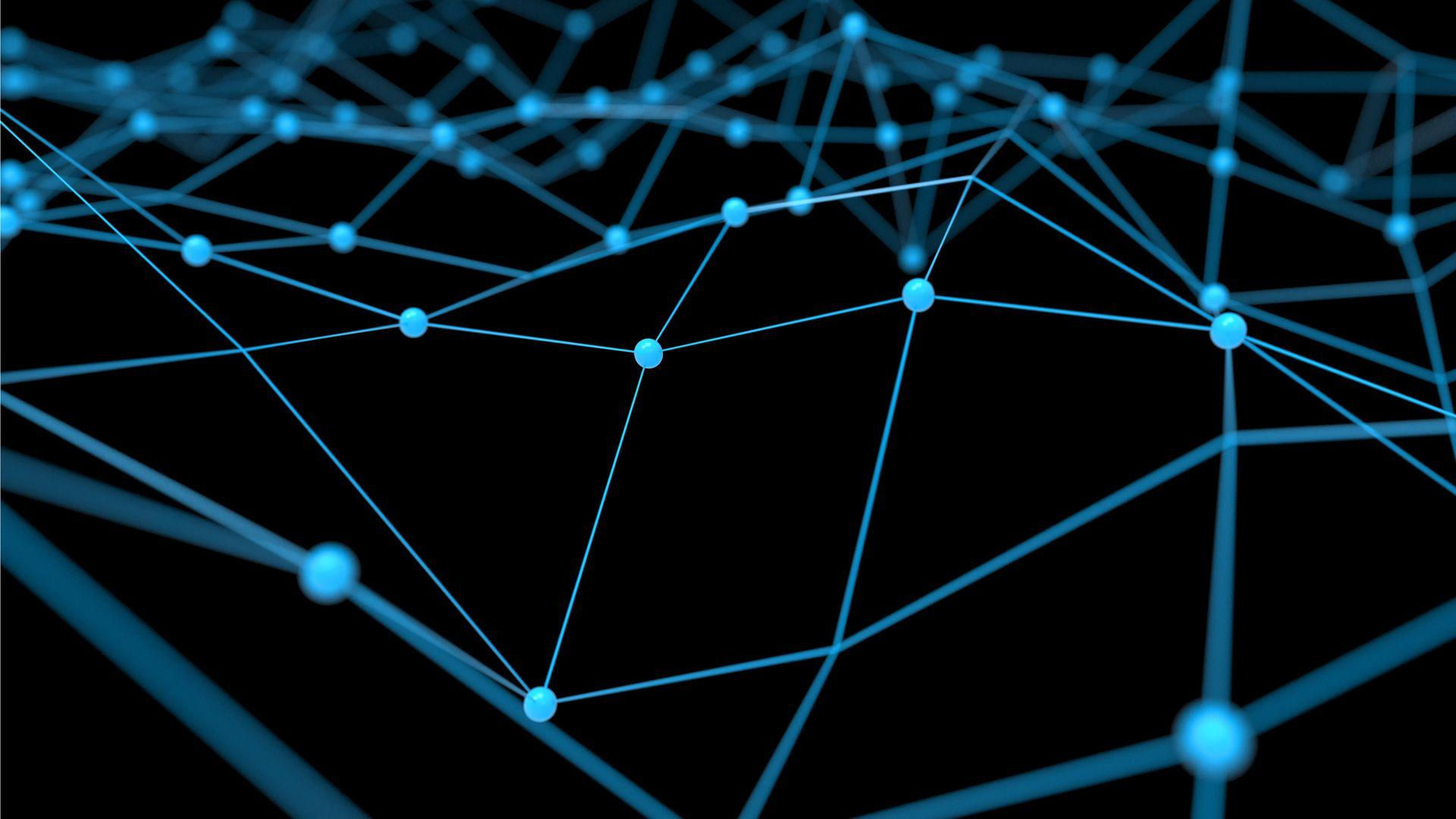
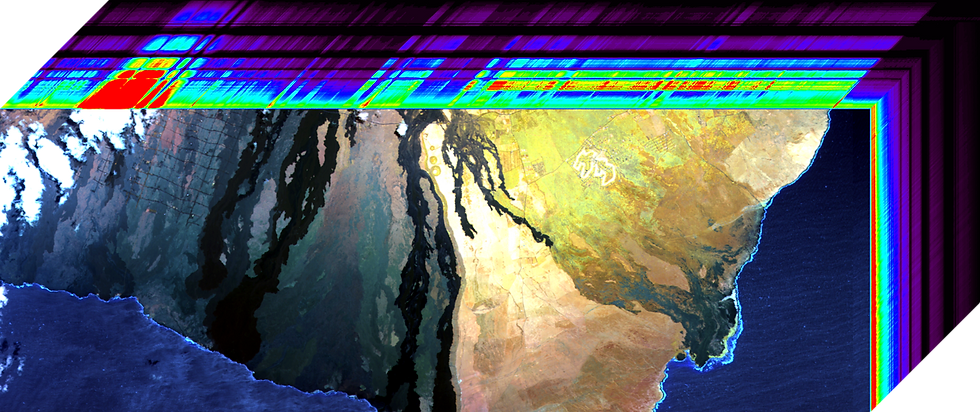
Hyperspectral Imaging
This is placeholder text. To change this content, double-click on the element and click Change Content.
I was interested during my International master internship in the processing of hyperpectral images. The work carried out concerns the linear unmixing of hyperspectral images considering an unsupervised scenario.
A hyperspectral image is obtained by considering the same scene observed at different wavelengths. By grouping all these images, we obtain a data cube that will be used for the analysis. Each pixel of this cube is represented by a spectrum whose number of samples corresponds to the number of wavelengths considered. Because of the low spatial resolution of these images, each pixel is represented by a mixture of several physical materials. The spectral unmixing appears then as a field in full expansion since it seeks to describe the interactions between the constituents of the imaged scene.
Bayesian non-parametric approach for linear spectral unmixing
We find several parametric Bayesian methods for linear unmixing in the literature. On the other hand, the number of articles on unmixing with nonparametric Bayesian methods is more limited. The work done during this internship consisted in proposing a new linear unmixing method based on a nonparametric Bayesian method. To do so, I started by studying and applying on synthetic data the different Dirichlet process mixture models (DPMM) using the three processes (Pólya's urn, Chinese restaurant and Indian bullet) that allow us to generate samples according to the Dirichlet process. Then, I chose the most suitable process in order to apply it to hyperspectral imaging for linear unmixing. The proposed algorithm will allow not only the estimation of the parameters (the abundances and the endmembers) but also their number. More precisely, a Gibbs sampler was used to generate samples asymptotically distributed according to the a-posteriori distribution of the different parameters. These samples were then used to estimate the different unknown parameters of the mixture model, via the Bayesian estimators MAP and MMSE. The resulting algorithm was validated on synthetic and real data.
213546
Power in Numbers
30
Programs
50
Locations
200
Volunteers
Project Gallery
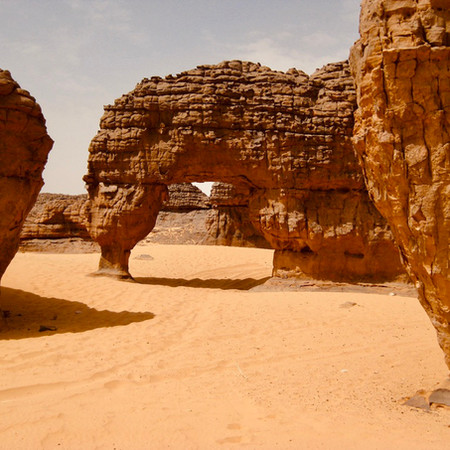
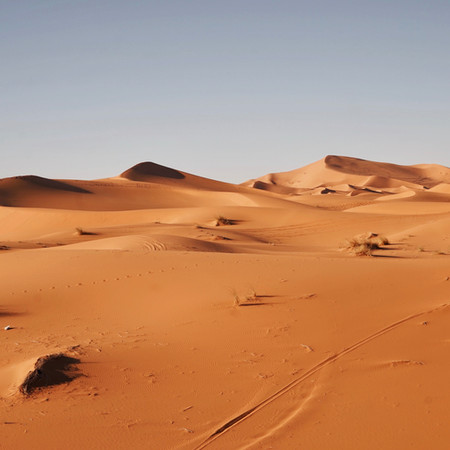
